Media
My fight against algorithmic bias
An essay by Robin Pocornie
Artificial intelligence (AI) is intended to make our lives easier. However, it often disadvantages certain groups – such as those from diverse racial and ethnic backgrounds, as well as individuals based on their sexual identity or age. This occurs when the data used in machine learning reflects existing societal biases. Robin Pocornie experienced this firsthand as a student. Since then, the now-scientist and speaker has been advocating for fairer AI-based systems. Here, she explains why more diverse datasets alone won't solve the problem.
At a glance:
-
Discriminated against by AI? Robin Pocornie made this experience by the use of facial recognition software.
-
Fighting for fair algorithms: Since then, she has been advocating for fair and just AI decision-making and calling for greater transparency in algorithmic decision systems.
-
Proposed solutions: Pocornie suggests a local data management, conducting bias audits, and shifting the focus from purely technological metrics to equity-centred metrics.
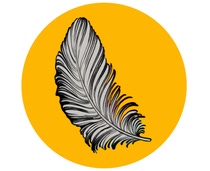
Facing unfair outcomes caused by racial bias
In 2020, at the height of the covid-19 pandemic, I became an AI justice advocate. Not by choice, nor due to my academic pursuits in computer science at the time, but because a mandatory facial detection algorithm failed to recognize my face. The online, at-home examination software implemented by my university was unable to detect students with darker skin tones. As a result, I faced delays and obstacles entering my exams, unlike my white peers. This compelled me to report my unfortunate and, one could say, hurtful experience to the university, especially as reports claimed more students worldwide were encountering the same issues.
After running through several hoops (and months) to find the correct university office to report the issue to, the university decided against honoring my request to cease the use of the online exam software. I have since made it my mission to fight for fair and just algorithms, and alter the way organizations, governments, and the private sector look at implementing these systems. In 2022 the Netherlands Institute for Human Rights ruled in an interim judgement that I had provided enough evidence to suggest algorithmic discrimination had occurred. However, after the university had provided counterevidence, the Institute concluded in October 2023 that although the specific software might be discriminatory, it had not been conclusively proven that discrimination took place in my particular experience.
The answer to fair and just algorithms does not only lie in more diverse datasets.“
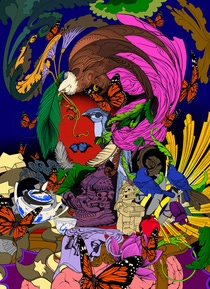
Datasets trained to establish our AI models have been shown to favor the reality of wealthy, European and North-American perspectives. This perpetuates race and gender stereotypes, which negatively affects systems combatting these inequalities. For example, text-to-image generators prompted to produce images of people in various occupations, yielded images of white males for high-income occupations and images of darker-skinned females in the low-income occupation category.
Additionally, AI can perpetuate gender bias in recruitment. This was exemplified by Amazon’s AI driven hiring model, which favored male candidates for technical roles due to historical data reflecting gender imbalances. This bias further highlights the need for transparency and fairness in AI systems to prevent discrimination and ensure equal opportunities for all candidates.
The prevalent solution proposed to address AI bias is to diversify the datasets used to train these systems. I believe that while these calls for more diverse datasets are well-intentioned, they are insufficient. We must adopt a different perspective that fundamentally rethinks AI development. This reimagination of AI development requires prioritizing justice and equity over mere technological capability.
3 Ways to fairer algorithmic decisions
I am often invited by companies and organizations to share my expertise on this ethical AI perspective. The solutions I propose to the growing bias in our decision-making models can be achieved at the developer’s desk and in the boardroom, as they take both the technological and organizational aspects of dealing with AI into account. What I find most important is to put visibility on the impact of AI, which often remains hidden from public view and can potentially create unforeseen ramifications.
1. Local data management
Firstly, empowering communities to collect, own and manage their own data can ensure that AI systems reflect more diverse realities. Localized data governance, where communities set the terms for data collection and usage, can prevent exploitation and misrepresentation.
2. Clear processes, regular audits
Secondly, implementing regular bias audits and establishing accountability mechanisms can address the ethical shortcomings of current AI systems. My case with the facial detection software is a prime example of this. If users cannot adequately escalate their (negative) experiences with AI, the entity that acquired and implemented it cannot be held accountable. This is especially problematic when these “system says no”-decisions cannot or can hardly be disputed.
3. New, fair criteria
Lastly, shifting the focus from purely technological metrics to equity-centred metrics can ensure that AI systems prioritize equity and fairness at the same level as the quantitative parameters. Examples of equity-centred metrices include fair working conditions at data centers, which often employ people from marginalized communities, and insight on environmental impact. The success of an algorithm should be measured not just by the direct output you see on the screen, but by its fairness, representation and proper consideration.
This approach requires a fundamental shift in how we think about AI, moving from a focus on technical prowess to include ethical responsibility and social justice. The way we build the system, and who benefits and is exploited in its development, is going to be extremely important.
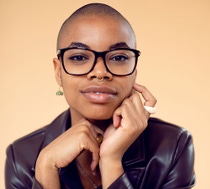
About the author
ROBIN POCORNIE
is a driven scientist and professional speaker in the field of technology and ethics and advises various organizations on the responsible use of algorithms. She is also the first person in the Netherlands to have generated case law on algorithms and discrimination.